Call for Papers: 4th Workshop on Fairness, Accountability, and Transparency in Machine Learning (FAT/ML 2017)
Halifax Regional Municipality
NS
Canada
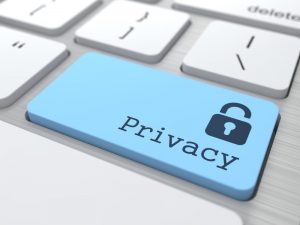
This workshop aims to bring together a growing community of researchers, practitioners, and policymakers concerned with fairness, accountability, and transparency in machine learning. The past few years have seen growing recognition that machine learning raises novel ethical, policy, and legal challenges. In particular, policymakers, regulators, and advocates have expressed fears about the potentially discriminatory impact of machine learning and data-driven systems, with many calling for further technical research into the dangers of inadvertently encoding bias into automated decisions. At the same time, there is increasing alarm that the complexity of machine learning and opaqueness of data mining processes may reduce the justification for consequential decisions to “the algorithm made me do it” or “this is what the model says.” The goal of this workshop is to provide researchers with a venue to explore how to characterize and address these issues with computationally rigorous methods. We seek contributions that attempt to measure and mitigate bias in machine learning, to audit and evaluate machine learning models, and to render such models more interpretable and their decisions more explainable.
This year, the workshop is co-located with the 23rd SIGKDD conference on Knowledge Discovery and Data Mining (KDD 2017). The workshop will consist of a mix of invited talks, invited panels, and contributed talks. We welcome paper submissions that address any issue of fairness, accountability, and transparency related to machine learning. This year, we place a special emphasis on papers describing how to bring tools for ensuring fairness, accountability, or transparency into real world applications of machine learning. We especially welcome submissions from practitioners in industry, government, and civil society.